Artificial Intelligence
AI hardware assistants leave a lot to be desired.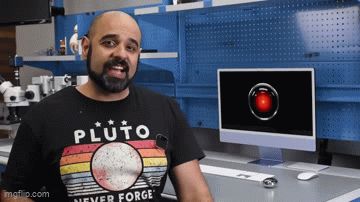
Artificial Intelligence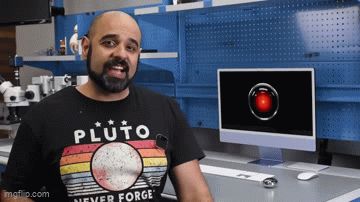
Should You Opt For An AI Hardware Assistant?Should You Opt For An AI Hardware Assistant?
These devices can provide instant AI-generated feedback, but does that convenience justify their added price and poor repairability?
Sign up for the Design News Daily newsletter.