Machine-Learning Research from Stanford, Toyota Bolsters EV Battery Design
New software can reduce the amount of testing and evaluation needed during the design process to hasten development.
March 27, 2020
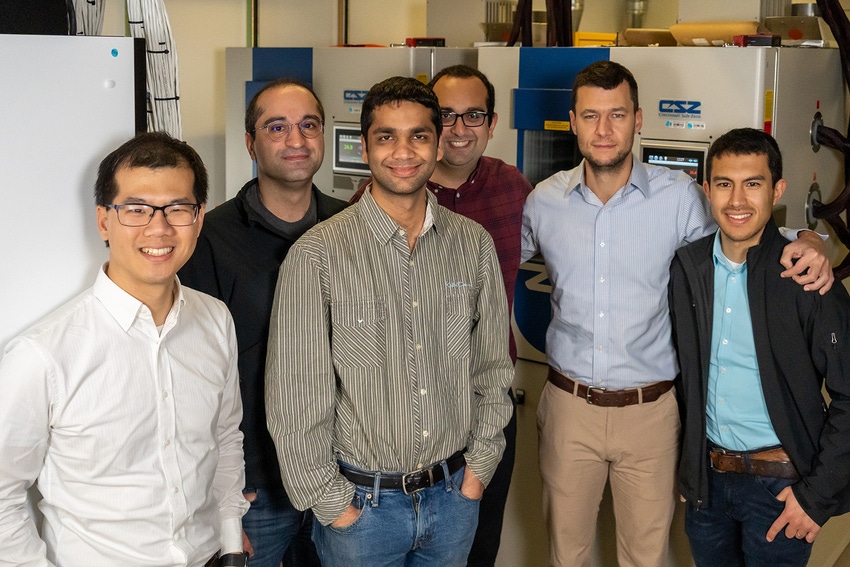
As electric vehicles (EVs) become a real alternative to cars that use fossil fuels for power, researchers continue to work to improve the performance of EV batteries.
To this end, a team of researchers at Stanford University—working with automobile manufacturer Toyota through the Toyota Research Institute—has developed a method of machine learning that can test batteries based on performance during the development process.
From left, the team that created a new machine-learning technique to reduce the time it takes to design EV batteries: Stanford Professor William Chueh, Toyota Research Institute scientist Muratahan Aykol, Stanford PhD student Aditya Grover, Stanford PhD alumnus Peter Attia, Stanford Professor Stefano Ermon and TRI scientist Patrick Herring. (Image source: Farrin Abbott) |
The new technique—invented by a team led by Stanford professors Stefano Ermon and William Chueh—can significantly reduce the amount of time it takes to test batteries, paving the way for more efficient development of longer-lasting, faster-charging EV batteries.
Chueh said the impetus for his team to develop the method was the current pace of research and development in the field of creating new EV technologies, which he said is quite slow. This is due to the evaluation time it takes to evaluate EV batteries during the development process, which can take months or even years to determine how long the devices will last, Chueh said.
“We were inspired by the pace of R&D for battery technology, which typically takes several years per year,” he told Design News. “This pace is dominated by both the long lifetime of batteries as well as the large design space that must be explored.”
Cutting Development Time
To hasten development by reducing evaluation times, the team created a software program that, based on only a few charging cycles, can predict how batteries would respond to different charging approaches, Chueh told us.
“We have developed a machine-learning platform which substantially reduces battery optimization time by predicting battery lifetime using minimal testing data and by intelligently exploring the design space--specifically, avoiding part of the design space that generates undesired results,” he explained to Design News.
The software also can decide in real time what charging approaches to focus on or ignore, which means it minimizes both the length and number of trials a battery must go through, Chueh said. Using their technique, researchers managed to cut the testing process from almost two years to 16 days.
While the team tested their method on battery charge speed, researchers said it can be applied to numerous other parts of the battery development pipeline and even to non-energy technologies.
Researchers published a paper on their work in the journal Nature.
Multi-faceted Approach
The team eyes using its approach to accelerate nearly every aspect of the battery-development pipeline: from design to form factor to the manufacturing process.
“Developing batteries that can meet multiple metrics simultaneously, such as energy density, fast charging, and safety, is challenging,” Chueh acknowledged. “In this work, we demonstrate that it’s possible to optimize batteries for fast charging--approaching the refueling time of gas cars--requiring 30 times less time compared to conventional optimization approaches.”
Researchers also believe their work can broad implications not only for EVs but for other types of energy storage, even in the area of developing storage solutions that can expand the use renewable energy like solar and wind on a global scale.
Elizabeth Montalbano is a freelance writer who has written about technology and culture for more than 20 years. She has lived and worked as a professional journalist in Phoenix, San Francisco and New York City. In her free time she enjoys surfing, traveling, music, yoga and cooking. She currently resides in a village on the southwest coast of Portugal.
About the Author(s)
You May Also Like