Researchers Teach Robots to Think Like Humans
Japanese scientists demonstrate how physical reservoir computing can be used to stimulate brain cells to control an AI machine.
November 9, 2021
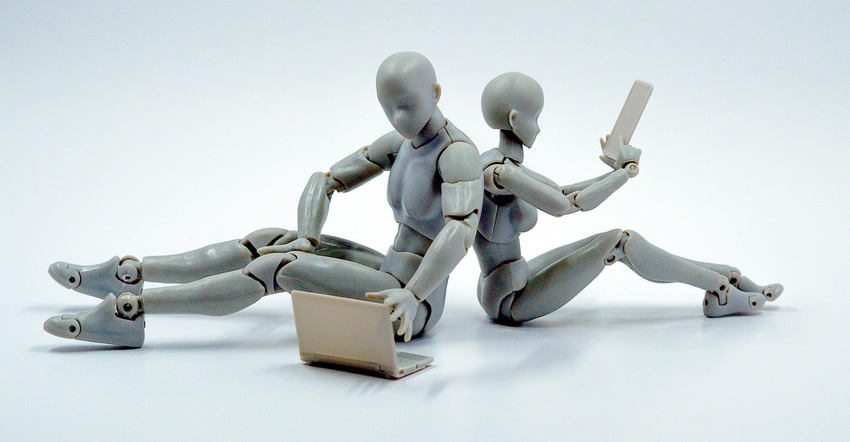
Visions of a future with robots that are as smart as or even smarter than humans are already being played out in science fiction films. Now scientists in Japan recently made strides in real life in teaching robots to think like humans using a new computing-based method.
A team of researchers from the University of Tokyo worked with an emerging technology called physical reservoir computing to create a link between a neural system and a machine. Specifically, researchers electrically stimulated a culture of brain nerve cells connected to an artificial intelligence (AI) machine to give it commands to follow, they said.
Using the technique, researchers demonstrated how a robot could be taught to navigate through a maze by electrically stimulating the brain nerve cells to which they were connected using first-order reduced and controlled error (FORCE) learning. They outlined their work in a paper published in Applied Physics Letters.
“Physical reservoir computing (PRC) is an emerging concept in which intrinsic nonlinear dynamics in a given physical system … are exploited as a computational resource or a reservoir,” researchers explained in an abstract for the paper. “Recent studies have characterized the rich dynamics of spatiotemporal neural activities as an origin of neuronal computation, sometimes as a reservoir, and demonstrated PRC in living neuronal cultures.”
Still, scientists have found it difficult to generate a coherent signal output from a spontaneously active neural system using PRC, which is where FORCE came into play in their work, according to the paper.
Neuro-Physical Link
Researchers used nerve cells, or neurons, grown from living cells to act as the physical reservoir for the computer to construct coherent homeostatic signals that told the robot the internal environment was being maintained within a certain range. They also acted as a baseline as it moved freely through the maze.
Whenever the robot steered itself in the wrong direction or faced the wrong way, researchers disturbed neurons in the cell culture with an electric impulse. The robot was continually fed the homeostatic signals interrupted by the disturbance signals until it successfully navigated through the maze, researchers said.
Throughout the experiment, the robot could not “see” or sense its environment and thus was entirely dependent on the electrical trial-and-error impulses. The findings suggest that using PRC, scientists can generate goal-directed behavior in robots without the need for additional learning using these disturbance signals, they said.
Moreover, based on this principle, physical reservoir computers can produce intelligent task-solving abilities, ultimately creating a reservoir that understands how to solve a task and thus creating an artificial way for robots to “think,” they said.
“A brain of [an] elementary school kid is unable to solve mathematical problems in a college admission exam, possibly because the dynamics of the brain or their ‘physical reservoir computer’ is not rich enough,” explained Hirokazu Takahashi, an associate professor of mechano-informatics at the university and a co-author of the paper, in a press statement. “Task-solving ability is determined by how rich a repertoire of spatiotemporal patterns the network can generate.”
Ultimately, researchers believe their use of physical reservoir computing in this context will lead to a better understanding of how the brain works and contribute to the development of neuromorphic computing systems.
Elizabeth Montalbano is a freelance writer who has written about technology and culture for more than 20 years. She has lived and worked as a professional journalist in Phoenix, San Francisco, and New York City. In her free time, she enjoys surfing, traveling, music, yoga, and cooking. She currently resides in a village on the southwest coast of Portugal.
About the Author(s)
You May Also Like