Researchers Have Taught Robots Self Awareness of Their Own Bodies
Columbia University researchers have developed an AI model that lets robots learn to model their own kinematics.
March 14, 2019
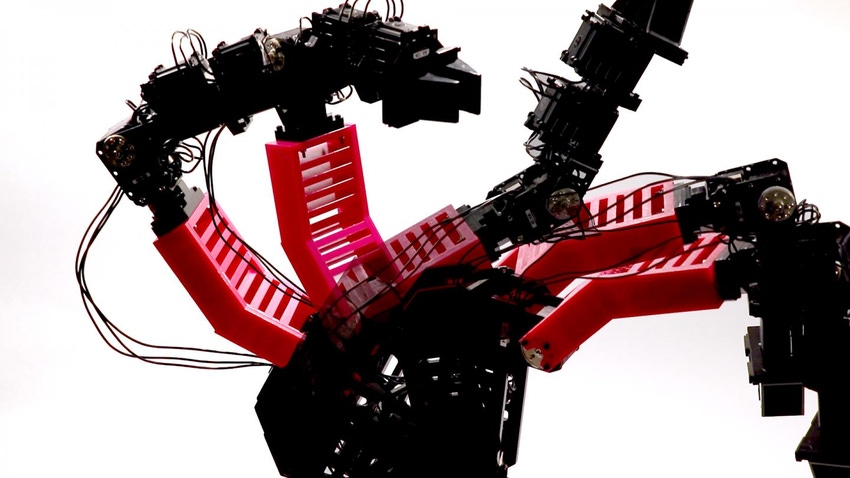
Columbia Engineering's robot learns what it is, with zero prior knowledge of physics, geometry, or motor dynamics. After a period of "babbling," and within about a day of intensive computing, the robot creates a self-simulation, which it can then use to contemplate and adapt to different situations, handling new tasks as well as detecting and repairing damage in its body. (Source: Robert Kwiatkowski/Columbia Engineering) |
What if a robot had the ability to become kinematically self-aware, in essence, developing its own model, based on observation and analysis of its own characteristics?
Researchers at Columbia University have developed a process in which a robot "can auto-generate its own self model," that will accurately simulate its forward kinematics, which can be run at any point in time to update and essentially calibrate the robot as it experiences wear, damage, or reconfiguration – thereby allowing an autonomous robotic control system to achieve the highest accuracy and performance. The same self model can then be used to learn additional tasks.
For robots designed to perform critical tasks, it is essential to have an accurate kinematic model describing the robot's mechanical characteristics. This will allow the controller to project response times, inertial behavior, overshoot, and other characteristics that could potentially lead the robot's response to diverge from an issued command, and compensate for them.
The robotic arm in multiple poses as it was collecting data through random motion. (Image source: Robert Kwiatkowski/Columbia Engineering) |
This requirement presents several challenges: First, as robotic mechanisms get more complex, the ability to produce a mathematically accurate model becomes more difficult. This is especially true for soft robotics, which tend to exhibit highly non-linear behavior. Second, once in service, robots can change, either through wear or damage, or simply experience different types of loads while in operation. Finally, the user may choose to reconfigure the robot to perform a different function from the one it was originally deployed for. In each of these cases, the kinematic model embedded in the controller may fail to achieve satisfactory result if not updated.
According to Robert Kwiatkowski, a doctoral student involved in the Columbia University research, a type of "self-aware robot," capable of overcoming these challenges was demonstrated in their laboratory. The team conducted the experiments using a four-degree-of freedom articulated robotic arm. The robot moved randomly through 1,000 trajectories collecting state data at 100 points along each one. The state data was derived from positional encoders on the motor and the end effector and was then fed, along with the corresponding commands, into a deep learning neural network. “Other sensing technology, such as indoor GPS would have likely worked just as well,” according to Kwiatkowski.
RELATED ARTICLES:
One point that Kwiatkowski emphasized was that this model had no prior knowledge of the robot's shape, size, or other characteristics, nor, for that matter, did it know anything about the laws of physics.
Initially, the models were very inaccurate. "The robot had no clue what it was, or how its joints were connected." But after 34 hours of training the model become consistent with the physical robot to within about four centimeters.
This self-learned model was then installed into a robot and was able to perform pick-and-place operations with a 100% rate in a closed-loop test. In an open loop test, which Kwiatkowski said is equivalent to picking up objects with your eyes closed (a task even difficult for humans), it achieved 44% success.
Overall, the robot achieved an error rate comparable to the robot's own re-installed operating system. The self-modeling capability makes the robot far more autonomous,Kwiatkowski said. To further demonstrate this, the researchers replaced one of the robotic linkages with one having different characteristics (weight, stiffness, and shape) and the system updated its model and continued to perform as expected.
This type of capability could be extremely useful for an autonomous vehicle that could continuously update its state model in response to changes due to wear, variable internal loads, and driving conditions.
Clearly more work is required to achieve a model that can converge in seconds rather than hours. From here, the research will to proceed to look into more complex systems.
RP Siegel, PE, has a master's degree in mechanical engineering and worked for 20 years in R&D at Xerox Corp. An inventor with 50 patents and now a full-time writer, RP finds his primary interest at the intersection of technology and society. His work has appeared in multiple consumer and industry outlets, and he also co-authored the eco-thriller Vapor Trails.
ESC BOSTON IS BACK! The nation's largest embedded systems conference is back with a new education program tailored to the needs of today's embedded systems professionals, connecting you to hundreds of software developers, hardware engineers, start-up visionaries, and industry pros across the space. Be inspired through hands-on training and education across five conference tracks. Plus, take part in technical tutorials delivered by top embedded systems professionals. Click here to register today! |
About the Author(s)
You May Also Like