Predictive Maintenance for People. Using AI to Prevent Suicide
In the face of a trend of Facebook suicides, researchers believe machine learning can help identify at-risk individuals on social media.
May 26, 2017
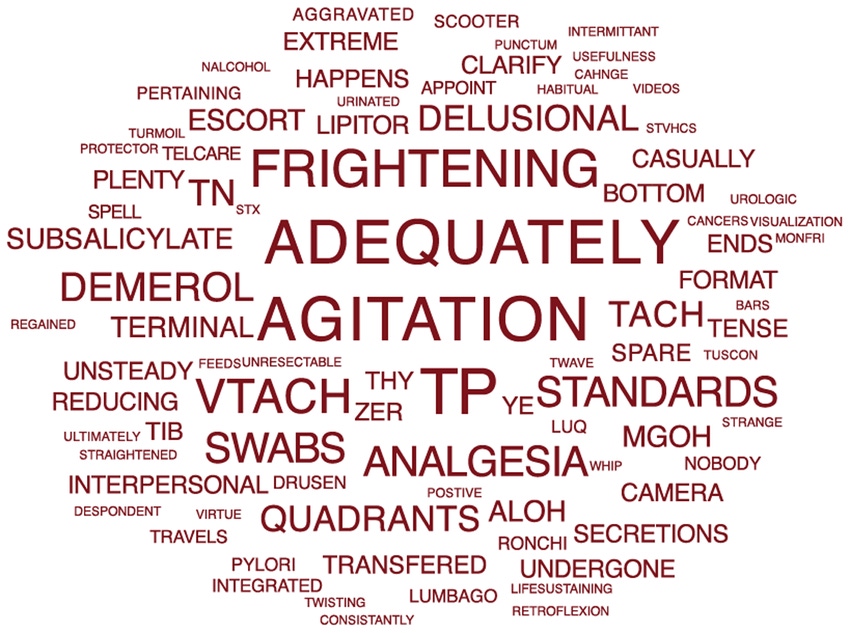
Since rolling out its Facebook Live feature the social network has seen a disturbing trend of people live-streaming their suicides. The very month Facebook debuted the live service 14-year-old Nakia Venant live-streamed her suicide. Most recently this month 33-year-old Jared McLemore of Memphis set himself on fire before running into a crowded bar where his ex-girlfriend worked.
The rash of incidents has raised such an outcry that Facebook CEO Mark Zuckerberg was forced to address it during his keynote at Facebook's F8 developer conference in April. Earlier this month, in a post via Facebook, Zuckerberg announced that the company would be bringing in an additional 3,000 members to its community operations team over the next year (bringing the total number to 7,500) in order to review content on Facebook and work with community groups and law enforcement to help them intervene if it appears a user is in danger from themselves or someone else.
For some the idea of hiring so many new people was not wrong, but is odd for a company so focused on leveraging machine learning and artificial intelligence (AI). An article in New York Magazine about the hirings reads: “Now, Facebook is bringing in 6,000 eyeballs to answer tough questions that computers can’t yet address, like, 'Is this footage of a person getting shot?' ”
Durkheim Project researchers created a machine learning algorithm capable of recognizing a patient at risk for suicide based on key words and phrases in clinical notes. (Image source: PLOS One / The Durkheim Project). |
But what if AI and machine learning could help with suicide prevention? Speaking at the recent 2017 Embedded Systems Conference (ESC) Conference in Boston earlier this month, Chris Poulin, Principal Partner of Patterns and Predictions, a big data analytics consulting group, believes that someday it can. “We have the ability to perform predictive maintenance on machines; why can't we do the same for people?” Poulin asked.
Poulin is the director and principal investigator of the Durkheim Project, a research effort (being conducted with the support of Facebook) seeking to create real-time predictive analytics for suicide risk. The aim is to use mobile phone and social media data to help mental healthcare professionals detect and monitor behavior and communications that could indicate suicide risk.
Poulin told the ESC audience that his team is trying to apply mathematics to language in the same way that Moneyball applies it to sports. “With Moneyball you're using all of these analytics to look at your team over time and trying to tell if certain risk factors will indicate if the team will win,” he said. “Only in this case you're trying to keep the team alive.”
The Durkheim Project (named after Emile Durkheim, a 19th century French sociologist who pioneered work on suicide) is based off research conducted in 2013 by Poulin and his colleagues. In a study, “Predicting the Risk of Suicide by Analyzing the Text of Clinical Notes,” published in 2014 in the open access journal PLOS One, Poulin and his team generated models using patient records of 210 U.S. veterans taken from the U.S. Veterans Administration (VA). Following an observation period from 2011 to 2013 the researchers divided the patients into three groups of 70 patients each: veterans who committed suicide (cohort 1); veterans who used mental health services and did not commit suicide (cohort 2); and veterans who did not use mental health services and did not commit suicide (cohort 3). The researchers used the clinical notes to generate datasets of single keywords and multi-word phrases that were markers for suicide risk according to clinicians. Words like “agitation,” “frightening,” and “delusional” were among the most common.
Using these keywords and phrases the researchers constructed prediction models using a modified version of Moses, a machine learning algorithm that uses a combination of genetic programming and probabilistic learning for language translation. Essentially, Moses can learn to detect patterns in language. For their study the researchers taught the algorithm to look for word patterns in the patients' clinical notes, then based on what it saw asked it to figure out if a patient was from cohort 1, 2, or 3.
What they found was that their algorithm was consistently 65% accurate or better at inferring which cohort a patient was from based on clinical notes. “Our data therefore suggests that computerized text analytics can be applied to unstructured medical records to estimate the risk of suicide,” according to the study. “The resulting system could allow clinicians to potentially screen seemingly healthy patients at the primary care level, and to continuously evaluate the suicide risk among psychiatric patients.”
Poulin said the study was only phase one of the Durkheim Project. The next two phases involve actual implementation and then intervention. In a currently ongoing study, Durkheim project researchers are having veterans opt-in to use a suite of Facebook and iOS and Android device applications that monitors their online activity and uploads relevant content to an integrated digital repository that is being continuously updated and analyzed by machine learning. As it monitors all of this incoming data the algorithm will provide real-time monitoring of words and behavioral patterns and learn to look for things that have been statistically correlated with suicidal tendencies and at-risk behavior.
If successful the Durkheim Project could enable detection and intervention of suicide risk at a scale that would be otherwise unfeasible for clinicians. In the 2014 study Poulin writes that it could also help improve clinicians' understanding of suicidal risk factors. “Many medical conditions have been associated with an increased risk for suicide, but these conditions have generally not been included in suicide risk assessment tools,” he wrote. “These conditions include gastrointestinal conditions, cardiopulmonary conditions, oncologic conditions, and pain conditions. Finally, some research has emerged that links care processes to suicide risk.”
Of course there's a long road ahead before people will be able to opt-in to have Facebook or their mobile device monitoring them for at-risk activity. There's more research to be done and after that will come the long road to FDA approval so that actual diagnosis and intervention can be provided. In their original study the Durkheim researchers also notes that further research using a larger patient population as well as more complex linguistic analysis would be very helpful. But if successful one algorithm may someday do more for people at risk of suicide than 7,500 reviewers ever could.
2017 Call for Speakers Smart Manufacturing Innovation Summit at Atlantic Design & Manufacturing. Designed for industry professionals looking to overcome plant and enterprise-level manufacturing challenges using IT-based solutions. Immerse yourself in the latest developments during the two-day, expert-led Smart Manufacturing Innovation Summit. You'll get the latest on the factory of future including insights into Industrial IoT and IIoT applications, predictive maintenance, intelligent sensors, security, and harmonizing IT/OT. June 13-15, 2017. Register Today! |
Chris Wiltz is the Managing Editor of Design News.
About the Author(s)
You May Also Like