Novel Way to Avoid Defects in 3D Printed Metal Parts
Making the connection between temperature and porosity in 3D printing could create more stable objects.
September 21, 2020
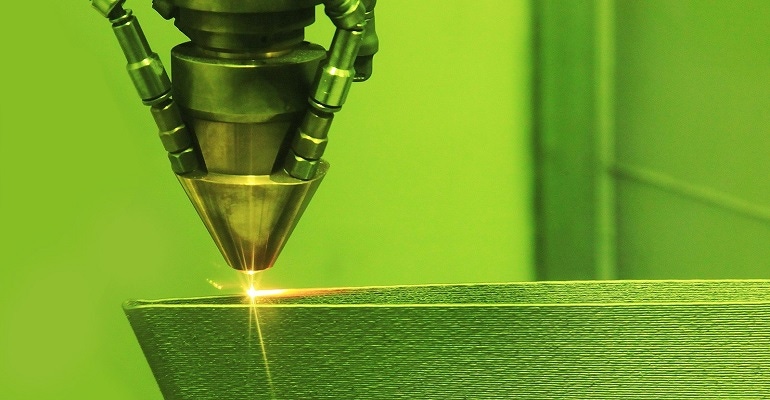
Researchers have been searching for various ways to control defects in the 3D printing process that can create parts and objects that are functionally compromised.
The U.S. Department of Energy’s (DOE) Argonne National Laboratory has teamed with Texas A&M University to develop a solution that uses temperature and its relationship to the stability of an object to help solve this problem in the printing of metal objects.
The team’s research suggests that by using temperature data at the time of production, those overseeing the additive-manufacturing process can predict the formation of subsurface defects in metal parts so they can be addressed on the spot, said Aaron Greco, group manager for Argonne’s Interfacial Mechanics & Materials group in the Applied Materials Division (AMD).
“Ultimately you would be able to print something and collect temperature data at the source and you could see if there were some abnormalities, and then fix them or start over,” he said in a press statement. “That’s the big-picture goal.”
Controlling Porosity
The key issue with defects in laser-based additive manufacturing processes is porosity within the object that forms while printing. Porosity refers to tiny, often microscopic “voids” that can occur that make the printed part prone to cracking and other failures.
The team used X-ray technology to design an experimental set-up allowing them to capture temperature data from a standard infrared camera viewing the printing process from above while they simultaneously used an X-ray beam taking a side-view. This gave them a comprehensive view of the identification of porosity forming below the surface of metal parts.
One phenomenon observed is thermal histories of objects in which the peak temperature is low and followed by a steady decline, a sign that correlates to low porosity. In contrast, thermal histories that start high, drop, and then later increase are more likely to indicate large porosity.
To make these kinds of connections, the team used machine learning algorithms to interpret data and predict the formation of porosity from the thermal history.
The results of this experimentation found that there is indeed a correlation between surface temperature and the potential for and existence of porosity, which is a start on the path of learning how to avoid it and thus potential defects.
Scaling the Process
The connection researchers made should help mitigate the risk associated with 3D printing errors in metal part production, which leads to cost for manufacturers, thus inhibiting widespread adoption of the technology, Greco said.
“To realize its full potential, we need to lower the risk to lower the cost,” he said in a press statement.
To drive the most and labor of the process down, researchers used 200 data points rather than millions that can be used in large-scale computer modeling to develop a “custom approach that made the best use of limited data,” said Noah Paulson, an AMD computational materials scientist at Argonne, in a press statement.
Researchers published a paper detailing their research in the journal Additive Manufacturing.
The team hopes future manufacturers can use the process to identify and correct defects at the time of printing because it would eliminate the need for costly and time-consuming inspections of each mass-produced component that exists today.
However, the work so far is just a “very, very good first step,” and the process must eventually be scaled to use thousands and thousands of data points required to build accurate models, Ben Gould, a materials scientist in the AMD, noted in a press statement.
Elizabeth Montalbano is a freelance writer who has written about technology and culture for more than 20 years. She has lived and worked as a professional journalist in Phoenix, San Francisco, and New York City. In her free time, she enjoys surfing, traveling, music, yoga, and cooking. She currently resides in a village on the southwest coast of Portugal.
About the Author(s)
You May Also Like