Preventive Maintenance Is Improved Through Predictive Analytics
With predictive analytics, a machine’s system efficiency and condition can be directly monitored using a variety of non-invasive measuring instruments.
January 10, 2019
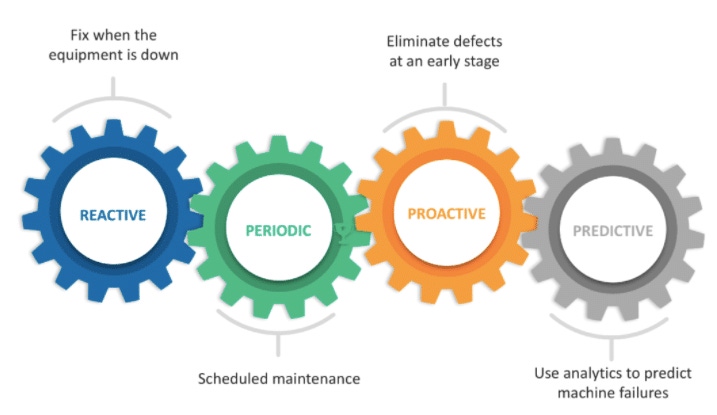
Traditionally, preventive maintenance (PM) relied on industrial or in-plant average life statistics, such as mean-time-to-failure (MTTF), to assist in the scheduling of maintenance events. With PM, a service log served as the communication instrument alerting plant or industrial maintenance technicians of machine breakdowns occurring over time. The log required documenting detailed information on the machine and its breakdown conditions by the plant or industrial maintenance technicians. Although the process was effective in recording the occurrence after the repair, machine breakdowns were still quite costly.
Today, however, maintenance activities can be effectively minimized by using predictive analytics (PA). With PA, a machine's system efficiencies and electrical and mechanical conditions can be directly monitored using a variety of non-invasive measuring instruments.
What Is Predictive Analytics?
Industry 4.0, or the Industrial Internet of Things (IIoT), uses connective devices or machines to aggregate data to the cloud. With this aggregated data, statistical algorithms (SA) and machine learning (ML) techniques determine the device or industrial equipment’s future performance outcomes. These future outcomes are traditionally identified as the machine’s health or behavioral status. The primary objective of PA is to assess what will happen in the future based on previous or current knowledge and experiences. PA drives Preventive Maintenance (PdM) activity through data analytics and modeling techniques of ML. Fault detection is a critical PA concept in PdM, which is well accepted in Industry 4.0.
The most reliable predictor of faults is based on the prominent algorithm of Principle Component Analysis (PCA). PCA allows the analysis of aggregated data to predict a future fault within an industrial machine. With PCA, catastrophic machine failures can be avoided using this effective predictive algorithm.
Predictive Maintenance Tools
To collect factual data on an industrial machine’s mechanical or electrical condition, sensory-monitoring devices are required. The data provides information for scheduling maintenance events on the industrial machine. Such a schedule can minimize or possibly prevent electrical and mechanical breakdowns of the plant floor equipment. PdM can capture machine anomalies before they become serious. There are three predominant PdM categories with associated tools that can assist the industrial maintenance or plant technician in this data aggregation process:
1. Smart Sensors
Industry 4.0 is heavily dependent on electronic sensors for collecting industrial machine performance. Electronic sensors allow slight changes to be monitored using wireless communications such as BLE (Bluetooth Low Energy) or WiFi protocols. ABB’s Ability Smart Sensor allows monitoring of low voltage motors with a WiFi enabled unit. The Ability Smart Sensor can monitor the motor’s health parameters, such as vibration, bearing condition, and temperature.
2. Analytics and Monitoring Tools
Analytics and monitoring tools measure an industrial machine’s performance on a regular basis. System parameters are measured and tracked over time. The aggregated data allows corrective action to occur before a catastrophic failure occurs. The software used to collect the data can perform the analytics and predict appropriate repair cycles for the industrial equipment. A remote monitoring system developed by Fluke allows data logging of the health status of industrial machines. The Fluke Condition Monitoring tools allow capturing of data and making PdM decisions based on trendline analysis (Linear Regression ML). The data is aggregated from the condition monitoring tools to the cloud using Fluke’s gateway device and software.
3. Schedulers
With data aggregated to the clouds, PM scheduling activities can be developed. The PdM is a subset of the planned PM cycle. This data aggregation is accomplished based on IIoT electronic sensors, condition monitoring systems and ML algorithms. The goal of PdM is to monitor and act on the industrial equipment when necessary. Automation techniques used in schedulers help deploy PdM activities to the industrial maintenance or plant technician. The primary function of the scheduler tool is to provide maintenance analysis data based on the trends identified within the data collection software. The PM scheduler will then appropriate the resources necessary to carry out the planned maintenance of the industrial machine.
With the assistance of PA, PdM can help in minimizing machine downtime. Electronic sensors, condition monitoring, and scheduler systems aided by PCA and ML techniques can ensure significant cost savings within an industrial environment. Additional information on Fluke’s Condition Monitoring tools can be found on its website. The ABB AbilityTM Smart sensor information is available on the website as well.
Don Wilcher is a passionate teacher of electronics technology and an electrical engineer with 26 years of industrial experience. He has worked on industrial robotics systems, automotive electronic modules and systems, and embedded wireless controls for small consumer appliances. He's currently developing 21st century educational products focusing on the Internet of Things for makers, engineers, technicians, and educators. He is also a Certified Electronics Technician with ETA International and a book author.
About the Author(s)
You May Also Like