What do the Experts Say About AI in 2021?
AI and machine learning are finally beginning to come into their own. What trends should we expect to see in 2021?
January 5, 2021
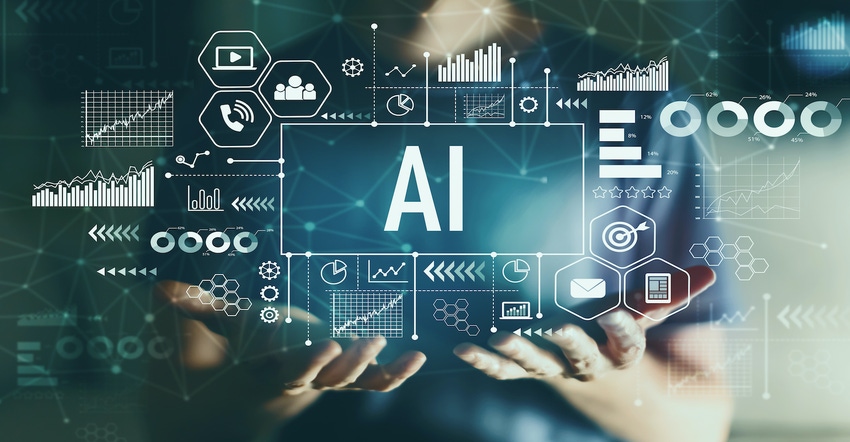
In 2012, researchers from the University of Toronto highlighted results at the annual ImageNet competition for computer vision systems that exceeded other contemporary programs by more than 10 percent. The technique used machine learning (ML) utilizing deep neural networks (deep learning) and marked the real start of artificial intelligence (AI) systems.
Fast-forwarding to 2020, both the commercial business and technical worlds have shown some real progress in the development of more complex AI systems. One example is the recent and well-received public offering of C3.ai in the enterprise space. Additionally, the technology has matured to the point that the US Air Force is using AI for the first time onboard a military aircraft. More generally, the use of AI for data analytics and predictive capabilities has been one of the major talking points in 2020 and will only increase in the coming years.
It’s important to remember that AI applies machine learning (ML), deep learning, and other techniques to solve actual problems. AI made the list of Gartner’s Top 10 Trends in Data and Analytics for 2020. This list cited the need for “smarter, faster, more responsible AI,” particularly those looking to “make essential investments to prepare for a post-pandemic reset.” In addition to the effects of COVID-19 on AI, the list underscored the importance of AI by predicting that, “by the end of 2024, 75% of enterprises will shift from piloting to operationalizing AI, driving a 5X increase in streaming data and analytics infrastructures.”
To gain a broader perspective on the benefits and challenges with the coming age of AI, Design News reached out to a range of experts in the electronics hardware and software industries to get their inputs on trends for 2021 and beyond. What follows is a portion of their insights.
AI used in speech recognition. |
Jem Davies, VP, Arm Fellow, and GM of Arm’s Machine Learning Group: Artificial intelligence (AI) and machine learning (ML) gain ground when their complexity gets pushed into the background. Over 1.5 billion people enjoy ML algorithms when they take smartphone pictures (or subsequently search for them in their ever-expanding photo files) generally without knowing it. The same phenomenon occurs whenever someone issues a command to one of the estimated 353 million smart speakers deployed worldwide.
Expect to see the invisibility spur the adoption of many applications. One-click smart parking will likely be the first experience with autonomous cars for many. Security systems that can accurately differentiate between the sound of a nearby prowler and a wandering raccoon will attract consumers.
Rich Fry, TDK Corporation of America: In 2021, we’ll continue to see governments, technology, and research institutions, and Information and communications technology or ICT companies looking even beyond 5G, meeting even future needs down the road as we look toward 6G (while simultaneously implementing and deploying 5G in the real world). The major MegaTrends advancing toward 6G include connected machines, the use of AI for wireless communication, more frequency and spectrum sharing, and openness of mobile communication.
Walden (Wally) C. Rhines, Ph.D. EE, CEO Emeritus of Mentor, a Siemens Business, and President and CEO, Corami: Artificial intelligence (AI) and machine learning (ML) processors will continue to be introduced to provide non-Von Neumann alternatives to machine learning. Meanwhile, Nvidia still dominates dedicated ML servers.
Chris Rowen, Ph.D. EE, CEO at BabbleLabs, Inc., former CEO of Tensilica: The process of mainstreaming AI into edge computing will continue to grow. New foundational capabilities in edge video processing will emerge to handle streams that are too high-bandwidth, too private, and too latency-sensitive to allow processing in the cloud. Conversely, deep-learning at the edge for lower bandwidth streams – like audio and sensor streams – will push the envelope more on functionality and less on raw compute levels. We expect to see widespread edge deployment of speech enhancement, recognition, and analytics-driven by improved latency, privacy, and cost.
Scott Rust, BS EE, Senior VP of Product R&D at NI: AI and ML systems will leverage exponential increases of computing horsepower and data storage to combine more data from more sources and in more new and novel ways. In 2021, we will see this continue to propel the state of the art and enable more accurate predictions from ML models.
Robert Ruiz, BS EE, Marketing Director, Hardware Analytics, and Test at Synopsys: In the coming year and beyond, test technology will continue its accelerated innovation pace, not just for manufacturing but also for new applications beyond manufacturing test. Recent, early adoption of a new testing paradigm utilizing high-speed I/O ports such as USB and PCIe marks the beginning of a trend to increase test data bandwidth dramatically. Such bandwidth is a game-changer, potentially decreasing silicon test time and test cost by an order of magnitude for next-generation AI and graphic processors. But perhaps even more exciting is how this new scheme, delivering massive test data on and off-chip, will enable other applications.
Richard Wawrzyniak, Principal Market Analyst, Semico Research Corp.: Since its appearance in 2012, the progress in the AI field has proceeded unabated at a sometimes-dizzying pace. The turnover rate for new innovations and architectural approaches is projected to be occurring every 3.5 months – much faster than in other periods of history in the semiconductor market. There are two main areas of focus:
-- Accelerators for data center applications: work continues to innovate new silicon architectures for domain-specific accelerators to aid in training for Deep Learning applications.
-- Inference architectures for Edge and End-Point silicon solutions: evolving market requirements for processing more data closer to the end application are pushing silicon architects to increase the CPU power and the compute resources to support these more powerful CPUs in end-point devices. In addition, the view of what Security functions are necessary is being recast towards embedding more powerful and robust security capabilities in these solutions.
Semiconductor fab workers manufacture AI chips. |
Emmanuel Sabonnadière, Ph.D., CEO of CEA-Leti: We expect quantum electronics, power-conversion devices, smart sensors, Edge AI (artificial intelligence), and green tech to be among the most vital – and competitive – R&D fields in microelectronics in 2021. The year will most certainly see an acceleration of the already-explosive growth in the number of connected objects, e.g. through the Internet of Things, which will turn into Intelligence of Things. This phenomenon will require new technologies to run Edge AI computation with very low energy consumption. We strongly believe in the short-term emergence of Edge AI, supported by neuro-inspired architectures and in-memory computing, which is a disruptive concept.”
Editor’s note: In 2019, CEA-LETI of France announced a key European AI collaboration with the Microelectronics Institute within Germany’s Fraunhofer Society. The two institutes’ work toward edge-AI systems is building up Leti’s strength in fully-depleted silicon-on-insulator (FD-SOI) chip design and the expertise of both Fraunhofer and Leti in 3D packaging. There is also the likelihood that it will draw upon finFET architectural research by another EU R&D powerhouse, Belgium’s Imec.
Omdia Analysts, Research and Report: Enterprise AI investments will expand in 2021 as AI moves towards scale and operationalization. Despite the COVID-19 crisis, 71% of respondents indicated they are “confident” or “very confident” that AI will deliver positive results during 2021 and beyond. Amidst market disruption, optimism remains for AI platforms and intelligent automation. Despite looming concerns among enterprise AI practitioners regarding scalability, speed-to-market, privacy, security, safety, and responsibility, there continues to be drive-in AI investment with focus turning to rigorous technologies like MLOps, and AI governance for more operational oversight.
Harvard Business Review: Integrating AI models into a company’s overall technology architecture has been a real problem. The missing component here is AI Operations — or “AIOps” for short. It is a practice involving building, integrating, testing, releasing, deploying, and managing the system to turn the results from AI models into desired insights of the end-users. At its most basic, AIOps boils down to having not just the right hardware and software but also the right team: developers and engineers with the skills and knowledge to integrate AI into existing company processes and systems. Evolved from a software engineering and practice that aims to integrate software development and software operations, it is the key to converting the work of AI engines into real business offerings and achieving AI at a large, reliable scale.
John Blyler is a Design News senior editor, covering the electronics and advanced manufacturing spaces. With a BS in Engineering Physics and an MS in Electrical Engineering, he has years of hardware-software-network systems experience as an editor and engineer within the advanced manufacturing, IoT and semiconductor industries. John has co-authored books related to system engineering and electronics for IEEE, Wiley, and Elsevier.
About the Author(s)
You May Also Like