Using Artificial Intelligence to Create Aperture Radar
Oculli has developed radar with the resolution to replace lidar in driver assistance systems.
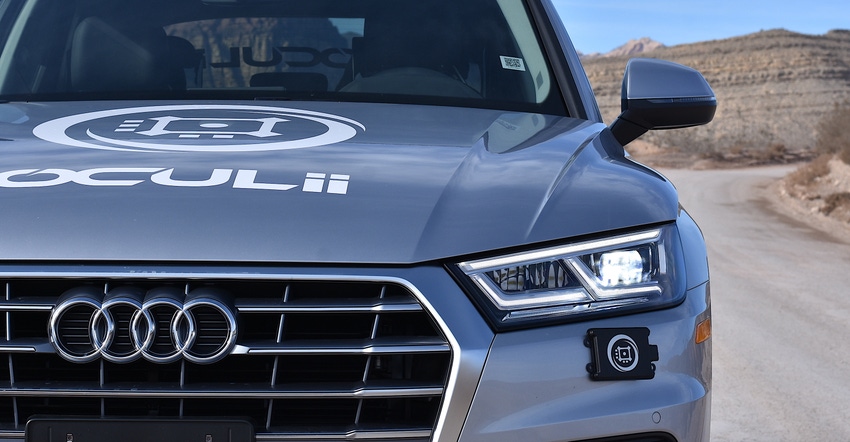
Carmakers are seeking to balance cost with capability as they increase the advanced driver assistance capabilities of their cars. Tesla, somewhat controversially, recently discontinued the use of radar on its vehicles in favor of a cameras-only sensor system. Oculli provides AI software for radar perception, so we thought it would be a good idea to talk to CEO and co-founder Steven Hong about his thoughts on what the tradeoffs are among camera, radar, and lidar systems and how smart radar can help fill in the gaps between their varying capabilities.
Design News: What are the pros and cons of radar sensors compared to cameras and lidar for advanced driver assistance systems (ADAS) systems?
Steven Hong: Radar is a durable, cost-effective, and market-proven technology that’s been around since the 19th century. It maintains its accuracy in poor weather conditions and has much lower power requirements compared to other sensors. The reason radar hasn’t been widely used in autonomous vehicles perception until now is because it had poor spatial resolution, limited sensitivity, and a narrow field of view.
But the newest radar sensors, particularly the ones we’ve built at Oculii, are equipped with AI software that allows them to learn from their environment, improving all those factors dramatically, as much as 100 times. Despite the better performance, the cost of these improved radar sensors is nearly equal to that of existing radar options, so for ADAS car manufacturers, there’s essentially no disruption to the manufacturing process.
Lidar and camera have both traditionally been better at sensing objects that are stationary or far away. Cameras in particular are good at seeing the world just as human eyes are designed to, which can be helpful when determining the words on a sign or the color of a stoplight.
They're also cheaper than lidar, which is one reason why Tesla is considering them. Their downside is that, just as human eyes, cameras can be fooled by shadows, bright sunlight, or the oncoming headlights of other cars. In addition, they have a hard time working if the lens gets dirty or is obstructed by rain or fog.
Historically, lidar has had the best accuracy of the three tools. It can perceive complex visuals such as the direction and speed of cyclists, and even predict their movement. That’s why many of the top-of-the-line autonomous vehicle producers use it. But for an ADAS system, that level of precision is not only overkill but is prohibitively expensive.
ADAS systems are produced for mass markets, and a lidar vehicle can cost as much as $70,000, not to mention the extra costs associated with storage and compute power. It’s orders of magnitude of cost that are simply unrealistic for the average ADAS mass-market vehicle producer.
DN: What is aperture imaging radar, and how does Oculii AI create a virtual version of that?
Steven Hong: Aperture imaging radar is a form of radar that is used to create two-dimensional images or three-dimensional reconstructions of objects. Typically, the way that reconstruction is achieved is by using an array of antennas to pick up radar waves. The radar wave bounces off of objects, and the antenna picks up those changes in wavelength to build a basic visual landscape.
The more antennas you have to collect that information, the clearer the image becomes. It’s a very cost-effective way to navigate, especially compared to lidars or cameras. The only problem is that to get the kind of image clarity and resolution you’d need to safely drive an autonomous vehicle, you’d need at a minimum, 200 antennae, and some of the latest radar systems out right now actually have that many. But each one of those antennas is going to increase cost, size, complexity, and power consumption.
What’s exciting about a virtual aperture imaging radar, like the one we’ve built at Oculii, is that you can use software to increase the performance of just a few antennae to get to a resolution you’d typically only get with hundreds. In our case, we use about twelve antennae per vehicle, but because that software is boosting the performance of each of those sensors and building a smarter conglomerate image, it’s essentially the same image clarity as a system with 200 antennas.
Even better, Oculii’s software platform evolves constantly by leveraging unique and proprietary radar data models. That means future generations of sensors built with Oculii AI software will scale exponentially, delivering significantly higher resolution and longer range in a cheaper and more compact package.
DN: What are the performance capabilities of Oculii virtual aperture imaging radar?
Steven Hong: Oculii has two products that deploy its virtual aperture imaging radar, EAGLE and FALCON.
EAGLE is the industry’s highest-resolution commercial 4D imaging radar. It can deliver joint 0.5-degree horizontal by 1-degree vertical spatial resolution across a wide 120-degree horizontal by 30-degree vertical instantaneous field of view. Generating radar images with tens of thousands of pixels per frame, EAGLE tracks targets at more than 350 meters, allowing the radar to operate in any type of weather, including rain, snow, or fog.
It’s also capable of precisely tracking targets that radar has a hard time perceiving, such as pedestrians and motorcyclists in crowded environments. That means an autonomous vehicle using EAGLE will have no problem navigating busy city streets. In addition, EAGLE delivers the spatial resolution of an 8-chip cascaded radar (24T32R) on a cost-effective, low-power 2-chip (6T8R) hardware platform. That means EAGLE consumes less than 5W of total power on a sensor that’s smaller than an index card.
Even smaller than that, FALCON is the industry’s most compact 4D imaging radar, delivering high-resolution performance in a 5cm x 5cm package that is smaller than a business card. Delivering 2-degree horizontal resolution across a wide 120-degree field of view FALCON can also simultaneously measure the elevation of the targets. With four FALCON sensors fused together, autonomous vehicles are provided with a 360-degree bubble of safety up to 200 meters in all directions that’s effective in all environmental conditions.
Built on a single-chip (3T4R), low-power, cost-effective hardware platform, FALCON consumes less than 2.5W of power, making it perfect for corner-radar automotive ADAS application or an autonomous robotic application where energy efficiency is critical.
DN: What are the potential cost savings of substituting this for lidar?
Steven Hong: It’s tempting to just look at the hardware costs of lidar versus radar, which when you analyze them, can be a difference of thousands of dollars. That’s already significant, but what’s even more significant when it comes to cost savings is what’s powering those two tools.
One the one hand, Oculii’s radar sensors are low-power, low-compute systems that place very little strain on the overall energy and data storage needs of a vehicle. On the other hand, the way lidar works means that it's placing a huge demand on the power consumption and data storage of an autonomous unit.
Lidar is a lot less efficient than radar because it generates millions of data points per second. That may sound great, but it actually means the computer system on an autonomous vehicle has to work extremely hard to actually store all those data points, sort out which ones matter and which ones don’t, and then interpret what that information actually means and what to do with it. All of that storage and computing is massively power hungry, delicate, and costly.
So hardware is one order of magnitude greater in terms of expense. But then data storage is another, and compute and processing is another. The base price tag of a lidar system, which is already pretty high, becomes exponentially more expensive when one factors in those additional components.
DN: Tesla has dropped radar from its cars in favor of a camera-only system. What is lost in terms of safety and reliability if other sensors are eliminated, as different sensors have different vulnerabilities, and multiple systems provide redundancy?
Steven Hong: What Tesla does with their camera system is effectively infer structure. If a human were to look out on a horizon and see three cars driving down the road, for example, they could not confidently claim that car A is 16.3 meters away and that car B is 85.2 meters away and that car is 100.7 meters away. You can get a feel of their relative distances but there’s no way of being able to tell for sure.
That’s what Tesla’s camera system is doing as well. It can approximate distance, but it can’t know for sure. And that might be ok for lower speed types of applications, but that inaccuracy at higher speeds and longer ranges could result in big issues.
Radar, and actually lidar as well, directly interrogate the environment and measure its 3D structure directly. Even if a camera is great at guessing, and is 99 percent accurate, radar and lidar both provide a direct measurement as a ground truth for the camera to confirm that its measurement is correct.
However, if the radar being used is very low resolution, that could actually start conflicting with what a camera is seeing. So as an example, say, a family of geese is crossing the road and the camera can see that but the radar, which isn’t good at picking up those small details, can’t. That radar and camera are suddenly telling the navigation system two different things: either there is something in the road or there’s not, and that’s going to confuse the system.
The radars that Tesla removed were very low resolution. They offered about a 10- or 15-degree resolution at about a 160-meter range. They were pretty low performance, as most traditional radars are. As a result, Tesla removing that radar sensor, in my opinion, actually doesn’t make that big a difference because what they were using was performing so poorly and actually was confusing its system. They also argue that their camera is so good it can replace lidar and all the cost and complexity associated with it.
But I believe that Tesla and others in the future will be using higher-performance radar sensors to enable level 3, 4, and 5 driving, because, in those instances, you do need redundancies in place for when a camera system breaks down for one reason or another. The improvements to radar that we’ve made at Oculii provide that backup just as accurately as a lidar system would but at a low cost and with very little change required to current manufacturing processes.
DN: How close is this technology to appearing on a production vehicle?
Steven Hong: We actually just announced our very first production vehicle partnership. Geely Motors, the owners of Volvo and the largest car manufacturer in China, just partnered with us to begin using our sensing technology in its British sports car brand, Lotus. The cars will use our EAGLE sensor, the highest resolution 4D imaging radar sensor on the market, starting between 2022 and 2023.
About the Author(s)
You May Also Like