Deepfakes: The Looming Threat Of 2020
Deepfakes have been lurking on the internet for years now. But in 2020 the AI technology will become a powerful weapon for misinformation, fraud, and other crimes.
January 6, 2020
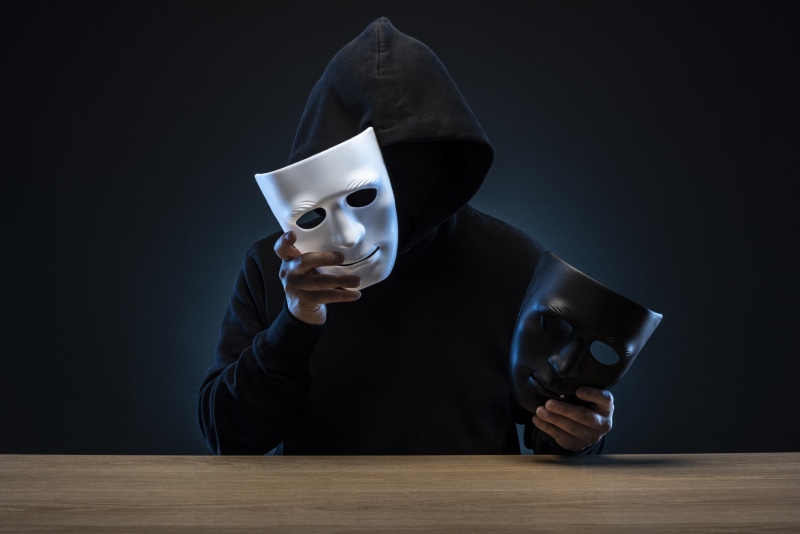
I'm watching a clip from the movie The Shining. Shelly Duvall is hiding from her crazed husband as he chops down the door with an axe. Jim Carrey sticks his head through the opening and cackles the iconic line: “Here's Johnny!”
...Jim Carrey is not in The Shining.
What you're seeing is not a Hollywood special effect. It wasn't done with After Effects, green screen, or with costuming and makeup. The video is a fake created by deep learning artificial intelligence – a deepfake. And anyone with a powerful computer and enough time can make one.
You might have heard of deepfakes before, or glimpsed headlines discussing the technology. You might even have laughed at various YouTube videos on channels such as Ctrl Shift Face that have swapped faces of celebrities in iconic roles to some humorous and sometimes unsettling results (once you've seen any of the bizarre deepfakes involving Nicolas Cage you can never un-see them.)
But deepfakes, once confined to darker corners of the internet, are becoming a serious threat. In the US, particularly as the 2020 election season rapidly approaches, AI experts are warning that deepfakes could become a powerful tool for spreading misinformation and manipulating the public. With enough effort a bad actor could create a video of any political candidate saying nearly anything. And in today's climate of social media outrage and algorithm-driven content distribution, there's no telling how far it could spread before someone caught it.
It's time engineers, developers, and technologists all had a serious discussion about deepfakes.
(Image source: Adobe Stock) |
The Origin Of Deepfakes
There's no one particular person that has taken credit for originally developing deepfakes. Their existence owes to a confluence of technologies ranging from ever-more sophisticated computer vision algorithms and neural networks, to increasingly powerful GPU hardware.
The first deepfakes to emerge on the internet seem to have emerged in 2017, when an anonymous Reddit user called “Deepfakes” began distributing illicit, altered videos of celebrities online. Other Reddit users followed suit and it wasn't long before a community had sprung up around distributing both deepfakes themselves as well as tutorials and software tools to create them.
In an interview with Vice, [NSFW link] one of the first outlets to take an extensive look at deepfakes, the Reddit user outlined how comparatively easy the process is:
“I just found a clever way to do face-swap. With hundreds of face images, I can easily generate millions of distorted images to train the network. After that if I feed the network someone else's face, the network will think it's just another distorted image and try to make it look like the training face.”
But it wasn't all fun and games. Far from it. When they first appeared, deepfakes had one particularly popular and disturbing use case – pornography. Much of the early deepfake content available was pornographic films created using the faces of celebrities like Gal Gadot, Scarlett Johansson, and Taylor Swift without their consent.
As the videos proliferated, there was an crackdown with Reddit itself shutting down its deepfakes-related communities, pornographic websites removing the content, and sites like GitHub refusing to distribute deepfake software tools.
If private citizens weren't that concerned yet it was probably because sites got somewhat ahead of the problem. Left unchecked it wouldn't have been long before deepfake pornography spread from celebrities to every day people. Anyone with enough publically available photos or video of themselves on a platform like Facebook or Instagram could potentially become a victim of deepfake revenge porn.
In 2018, Rana Ayyub, and investigative journalist from India, fell victim to a deepfakes plot intended to discredit her as a journalist. Ayyub detailed her ordeal in an article for The Huffington Post:
“From the day the video was published, I have not been the same person. I used to be very opinionated, now I’m much more cautious about what I post online. I’ve self-censored quite a bit out of necessity.
“Now I don’t post anything on Facebook. I’m constantly thinking what if someone does something to me again. I’m someone who is very outspoken so to go from that to this person has been a big change.
“I always thought no one could harm me or intimidate me, but this incident really affected me in a way that I would never have anticipated...
“...[Deepfakes] is a very, very dangerous tool and I don’t know where we’re headed with it.”
How Deepfakes Work
On the surface the process of creating a deepfake is fairly straightforward. First, you need enough images (hundreds or more ideally) of your target – showing their face in as many orientations as possible (the more images you can get, the better the results – hence why celebrities and public figures are an easy target). If you think it might be difficult to get hundreds or thousands of images of someone remember that a single second of video could contain 60 frames of someone's face.
Then you need a target video. The AI can't change skin tone or structure so it helps to pick a target and source with similar features. Once a deep learning algorithm is trained on a person's facial features, additional software can then superimpose that face onto another person's in your target video. The results can be spotty at times, as many videos online will attest to, but done right, and with enough attention to detail, the results can be seamless.
In an interview with Digital Trends, the anonymous owner of the Ctrl Shift Face YouTube channel (the channel responsible for the Jim Carrey/The Shining videos, among others) discussed how simple, yet time-consuming the process is:
“I’m not a coder, just a user. I don’t know the details about exactly how the software works. The workflow works like this: You add source and destination videos, then one neural network will detect and extract faces. Some data cleanup and manual extraction is needed. Next, the software analyzes and learns these faces. This step can sometimes take a few days. The more the network learns, the more detailed the result will be. In the final step, you combine these two and the result is your deepfake. There’s sometimes a bit of post-process needed as well.”
On one hand, the relative ease at which this can be done with little to no coding experience is certainly disconcerting. On the other however, deepfakes are an impressive demonstration of the sophistication of AI today.
At the core of deepfakes is a neural network called an autoencoder. Put simply, an autoencoder is designed to learn the important features of a dataset so it can create a representation of it on its own. If you feed a face into an autoencoder its job is then to learn the distinguishing characteristics that make up a face and then construct a lower-dimensional representation of that face – in this case called a latent face.
Deepfakes work by having a single encoder train to create a generalized representation of a face and then have two decoders share that representation. If you have two decoders – one trained on Person A's face, the other on Person B's – then feed the encoder either face you can transpose Person A's face onto Person B's (or vice versa). If the encoder is trained well enough, and the representation is generalized enough, it can handle facial expressions and orientations in a very convincing way.
Since faces in general are very similar in their overall shape and structure, a latent face created by an encoder using Person A's face, can be passed to a decoder trained on Person B's face to good effect. The result at the other end is a video of Person B, but with Person A's face.
As long as you have two subjects similar enough and a computer with enough processing power, the rest just takes time. Faceswap – one of the more readily available deepfakes apps – can run on a Windows 10, Linux, or MacOS computer and recommends a newer Nvidia GPU for processing. “Running this on your CPU means it can take weeks to train your model, compared to several hours on a GPU,” according to Faceswap's documentation.
Deepfakes Vs. 2020
Some of the first research to really sound the alarm bells about the political dangers of deepfakes appeared from the University of Washington in 2017. Using video footage of then-President Barack Obama, along with audio from one of his speeches, University of Washington researchers were able to create a realistic, synthesized video of Obama giving that speech.
“Trained on many hours of his weekly address footage, a recurrent neural network learns the mapping from raw audio features to mouth shapes,” the researchers wrote. “Given the mouth shape at each time instant, we synthesize high quality mouth texture, and composite it with proper 3D pose matching to change what [Obama] appears to be saying in a target video to match the input audio track. Our approach produces photorealistic results.”
For anyone paying attention the implications were clear. The University of Washington researchers had used audio from actual speeches. But what would happen if someone used doctored or fake audio? In 2018, filmmaker and comedian Jordan Peele (who does a great impression of Obama's voice) demonstrated just that in a PSA video created in collaboration with Buzzfeed to warn against the dangers of deepfakes:
“Deepfakes are going to be a greater challenge in 2020, and it will have a menacing effect on everything -- be it entertainment, or politics, or business,” Sandeep Dutta, chief practice officer, APAC, at Fractal Analytics, an AI analytics company, told Design News. “When we wake up in the morning, the first thing we do is scan through digital media to understand what is going around the world through apps like Facebook, etc. Over time, this has become habit forming and we seem to trust what we see and let it lead our thoughts and beliefs without knowing if it is real or malicious.”
Ben Lamm, CEO of Hypergiant Industries, a provider of AI products and consulting services, told Design News that the election year makes the impact of deepfakes particularly concerning. “In a time when facts and truth are at war with propaganda, groupthink, and disinformation, deepfake technologies pose a huge threat to the legitimacy of content,” he said. “At any given moment, over 30 countries are engaging in cyberwarfare. With this technology on-hand, false accusations and misrepresentations are provided with added ammunition beyond simply 'he said, she said.' They’re given a face and a personality.”
Lamm said he has no doubt that in 2020, deepfake videos will pose a very real threat and be used to attempt to divide the American people and influence voting behavior.
“It’s imperative that the government understands deepfake technology because of the outside impact on our political system, but also because it fundamentally impacts the fabric of our society,” Lamm said. “Concurrently, there is a strong need for more technology policy that regulates against the misuse of and creation of technologies that harm our society.”
While legislation can play a supporting role in combating deepfakes, for Dutta the solution will lie in more self-governance by the tech and media industry.
“Legislation will help. But, in the tech category, regulations lag behind,” Dutta said. “That is why we should not depend upon it. I don’t think that legislation, with the sophistication needed, will come in to tackle the problem. Regulators are not known to be tech-savvy or to work quickly. Even where there is regulation, enforcement will be a challenge.”
Fighting Fire With Fire
Companies are already developing algorithms capable of detecting deepfakes and other malicious content. Rochester,NY-based Blackbird.AI has made its mission to use “machine learning and interdisciplinary human intelligence to combat disinformation.” Blackbird.AI’s technology detects misinformation in real-time and at scale.
Brice Chambraud, managing director of Blackbird.AI in APAC, explained to Design News:
“ 'Deepfake' is starting to be used as a blanket term for altered video, images, and text. We built our architecture to first check for content that is synthetically amplified, for example, whether it is spread as part of a coordinated misinformation campaign, along with harmful content, then analyze that content for deepfakes.
“As deepfake detection is computationally heavy and requires significant resources, the benefits of our approach is a significant reduction in scale and the ability to capture all types of harmful content including deepfake videos, text based propaganda, and other doctored content.”
For Chambraud, deepfakes will be less of a concern in 2020 than disinformation from text and memes. He stresses the importance of tools to fight these things as well. “There are many other types of manipulated content already causing harm, more so text-based, AI-generated misinformation flooding the internet at scale, causing mass confusion,” he said. “Deepfake technology has yet to reach a stage used by threat actors at scale, but it remains one of the fastest progressing technologies and a major threat in the near future.”
“The same deep learning technology that is responsible for creating deepfakes can be used to cure it,” Dutta said. “Algorithms need to be robust and better designed to detect a real video versus a fake video or image at the source. For example, before somebody uploads a video on YouTube or Facebook, there needs to be a check to identify whether the video is real or fake. Deep learning tools can be made more sophisticated to identify inconsistencies at the pixel level with high precision. Research shows that three of the most common deepfake techniques are known as 'lip sync,' 'face swap,' and 'puppet master.' These techniques, however, can create a disconnect that may be uncovered by a clever algorithm as a way to combat deepfakes.”
That's not to say that developing anti-deepfake technologies is a quick and easy fix. “The problem here is one of iterations,” Lamm said. “Yes, GANs [generative adversarial networks] can help to combat deepfake tech. However, over time both systems will become better and better. The end result is a race to fight a technology in iterations. It’s not a magic bullet. It’s a Band-Aid.”
The Public (Deep) Face
Then what about the general public? Surely something that can create more funny videos of Nic Cage can't be all bad.
“Deepfakes can possibly add value in entertainment, helping creators enhance visual storytelling and provide immersive gaming experiences. However, this needs to be stewarded responsibly,” Blackbird.AI's Chambraud said.
“Of course there are potential upsides to deepfake tech, just as there is with any technology. No technology is inherently bad, but any technology can be used to do bad if applied in unethical ways. That said, we have to weigh the positives against the negatives and come up with unique, creative solutions from both technology and policy perspectives to address the negatives without hindering the positives,” Hypergiant's Lamm said.
“Generally, I do believe this is one tech development where the negatives outweigh the positives,” Fractal Analytics' Dutta said. “However, deepfakes can potentially offer an upside in personalization. For example, deepfake technology can be used for translating many different languages – allowing people to connect more across languages. On the business front, company CEOs and celebrity endorsers could speak directly to individuals with customized messages, even referring to a consumer by name. The third aspect is possibly recreating famous historical figures who have inspired us or left positive impacts on society. There may also be applications in education and running social programs.”
But even with those potential upsides, many experts agree that deepfakes could have much more of a downside than any lasting beneficial impact.
In an October 2019 blog, Jeff Pollard, VP and principal analyst at Forrester predicted that costs associated with deepfake scams – ranging from fraud to ransom attacks – will exceed $250 million in 2020. In August 2019 The Wall Street Journal reported on an incident in which cybercriminals were able to trick the CEO of a UK-based energy company into wiring them $243,000 by using deepfake software to mimic the voice of his boss over the phone.
“One of the reasons deepfake works so well is because a large portion of people are willing to believe them and spread them,” Dutta said. “Our society needs to be educated on what deepfakes can do as a technology and what one can do at their level to mitigate it.” He outlined some tell-tale signs of a deepfake video such as face discoloration, lighting changes, poor audio synchronization, and blurriness that people should watch out for.
“People need to understand this and learn to not trust everything that they see. They should be made aware of some of the basic tools that can be used for verification – like a reverse image search by uploading a video into the Google search bar.”
“The moment deepfake technologies can run efficiently and inexpensively in combination with text, audio, and images, extremely compelling websites can be developed and adversaries can create a huge amount of deceptive content and confusion,” Chambraud said. “With key insights on content from tools such as credibility labels, users can better understand if specific content is harmful and make informed decisions on the information they consume and share within their network. Over time we see this working like spam detection, whereby misinformation detection technologies will improve and people will be more comfortable accepting suggestions to ignore harmful manipulated content.”
From Lamm's perspective however, the challenge here is getting awareness to everyone – not just those with a vested or particular interest in ensuring and verifying that the information they consume online is real and factual. “Understanding what a deepfake is and how to spot one would be a great way to minimize the influence that they can have on people who specifically are interested in finding factual content, and have an active concern for deepfake influence themselves,” Lamm said. “Those who are not as proactive with their research into this and other technologies, as well as people who remain loyal to opinion over fact may not be as easy to educate. These are the people that would need the education the most, but you can’t teach someone who doesn’t want to be taught.”
The Reaction From Big Tech
For their part, the big tech companies have stepped up their fight against deepfakes. Google has made its own library of synthetic videos freely available to researchers can companies to use to help build deepfake identifying tools.
Facebook and Microsoft, meanwhile, have partnered with universities to conduct the Deepfake Detection Challenge (DFDC) with the aim of developing deepfake detection solutions to provide to the media industry. The DFDC gives participants a dataset consisting of real videos made with actors and doctored videos generated by AI. Facebook itself has pledged $10 million to fund the challenge.
Adobe has been working with researchers at UC Berkeley to develop AI that can distinguish real facial photos from fakes. As the company behind Photoshop, Adobe obviously has a vested interest in ensuring its software isn't being used with malicious intent.
“Technology companies need to start from a position of understanding intent: What are we developing, why and how might people use this to harm society?” Lamm said. “We are no longer willing to accept a 'do no evil' policy by technology companies – instead we must hold people to a standard to do good.”
Chaumbaud encourages a focus away from hardware and more toward the social platforms and channels that could be utilizing and distributing deepfakes. “At the very least, they need to be held accountable to measure and label the creation of disinformation on their platforms,” he said. “As a start, platforms that are currently utilizing video altering technologies should consider providing metadata and watermark content to make it easier to authenticate.”
“Big tech has to own the responsibility to counter [deepfakes] with better AI tech,” Dutta said. The floodgates are already open and as computer hardware continues to grow more powerful it's only going to enable more and more people to create deepfakes. Five to 10 years ago it would have been a pain to take video from your smartphone, put an animated graphic over it, and upload it online. Now it can all be done in seconds within apps themselves.
The same thing will happen for deepfakes. And when it does it's going to become very hard for any of us to believe anything we see or hear online. Snapchat is currently rolling out a new feature, Cameo, that uses deepfake-like technology to put a user's face into videos.
“The technology is already advancing by leaps and bounds and will further accelerate [in 2020], making the deepfakes even more sophisticated and difficult to catch,” Dutta said. “The discerning may be cautious, but the problem will be with the majority, where deepfakes will play to echo-chambers of their biases and deepen them.
There is no turning back this tide.”
RELATED ARTICLES:
Chris Wiltz is a Senior Editor at Design News covering emerging technologies including AI, VR/AR, blockchain, and robotics.
About the Author(s)
You May Also Like