AI Technique Aims to Prevent Medical Imaging Cyber Threats
Researchers at Ben-Gurion University of the Negev (BGU) have developed a new artificial intelligence solution that may protect medical devices from malicious operating instructions in a cyberattack, as well as from human and system errors.
October 1, 2020
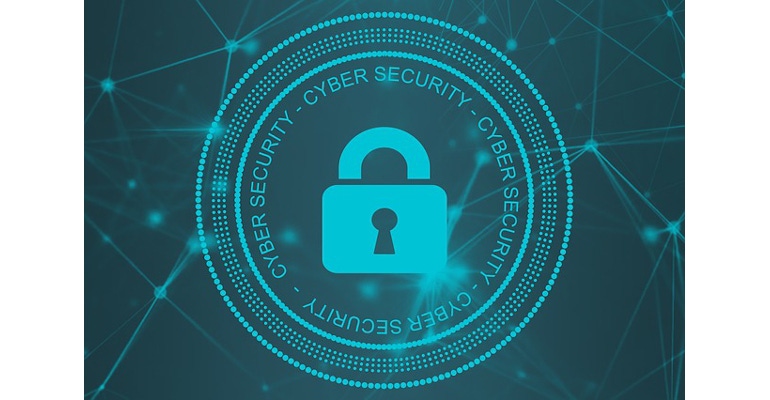
In May 2017, National Health Service (NHS) hospitals in England and Scotland were virtually shut down for several days because of the global WannaCry cyberattack. The attack resulted in the cancellation of thousands of appointments and operations and some NHS services had to turn away noncritical emergencies. Up to 70,000 devices, including computers, MRI scanners, blood-storage refrigerators, and operating room equipment may have been affected.
And in 2016, the Hollywood Presbyterian Medical Center in Los Angeles paid $17,000 in bitcoin to a hacker to unlock data that had been encrypted in an attack. Hospital staff struggled to deal with the loss of email and access to patient data. Some patients were transported to other hospitals because of the incident and computers essential for various functions, including CT scans, documentation, lab work, and pharmacy needs, were offline.
These events highlight how vulnerable medical devices can be to cyber threats. CT, MRI, and ultrasound machines are controlled by instructions sent from a host PC. If these instructions are abnormal or anomalous—because of cyberattacks, a technician’s configuration mistake, or host PC software bugs—potentially harmful threats to patients can occur, such as radiation overexposure, manipulation of device components, or functional manipulation of medical images.
Tom Mahler, a PhD candidate under the supervision of BGU Professors Yuval Elovici and Professor Yuval Shahar in the University’s Department of Software and Information Systems Engineering, has proposed an AI technique that may eliminate or reduce the potential for threat from these cyberattacks or other system or human errors.
Mahler’s approach uses AI to analyze the instructions sent from the PC to the physical components using a new architecture to detect anomalous instructions. The dual-layer architecture focuses on two types of anomalous instructions—context free (CF), which are unlikely values or instructions, such as giving one hundred times more radiation than typical—and context sensitive (CS), which are normal values or combinations of values, but are considered anomalous relative to a particular context, such as mismatching the intended scan type, or mismatching the patient’s age, weight, or potential diagnosis.
The research team evaluated the new architecture in the CT domain, using 8,277 recorded instructions and evaluated the CF layer using 14 different unsupervised anomaly detection algorithms. They evaluated the CS layer for four different types of clinical objective contexts, using five supervised classification algorithms for each context.
Adding the second CS layer to the architecture improved the overall anomaly detection performance from an F1 score of 71.6%, using only the CF layer to between 82% and 99%, depending on the clinical objective or the body part. The CS layer enables the detection of CS anomalies, using the semantics of the device’s procedure, an anomaly type that cannot be detected using only the CF layer.
The architecture could easily be incorporated into machines currently being used in healthcare settings. “One of the key problems medical device manufacturers have is that any update they make to the existing device must follow strict safety guidelines,” Mahler explained to MD+DI. “This is an expensive and time-consuming process that makes updating existing devices not practical. With our dual-layer architecture, it is possible to plug it into an existing device to make it secure.”
Mahler said that it is possible to run the analysis in parallel to the CT scan. “Even if the scan is already started, if an error is detected, the technician can quickly halt the device operation even a few seconds into the scan,” he explained.
For now, Mahler plans to continue researching and improving this method. He presented his research, “A Dual-Layer Architecture for the Protection of Medical Devices from Anomalous Instructions,” at the 2020 International Conference on Artificial Intelligence in Medicine (AIME 2020).
About the Author(s)
You May Also Like